Riding the AI Wave in Real Estate: Insights from McKinsey’s Latest Analysis
Nov 17, 2023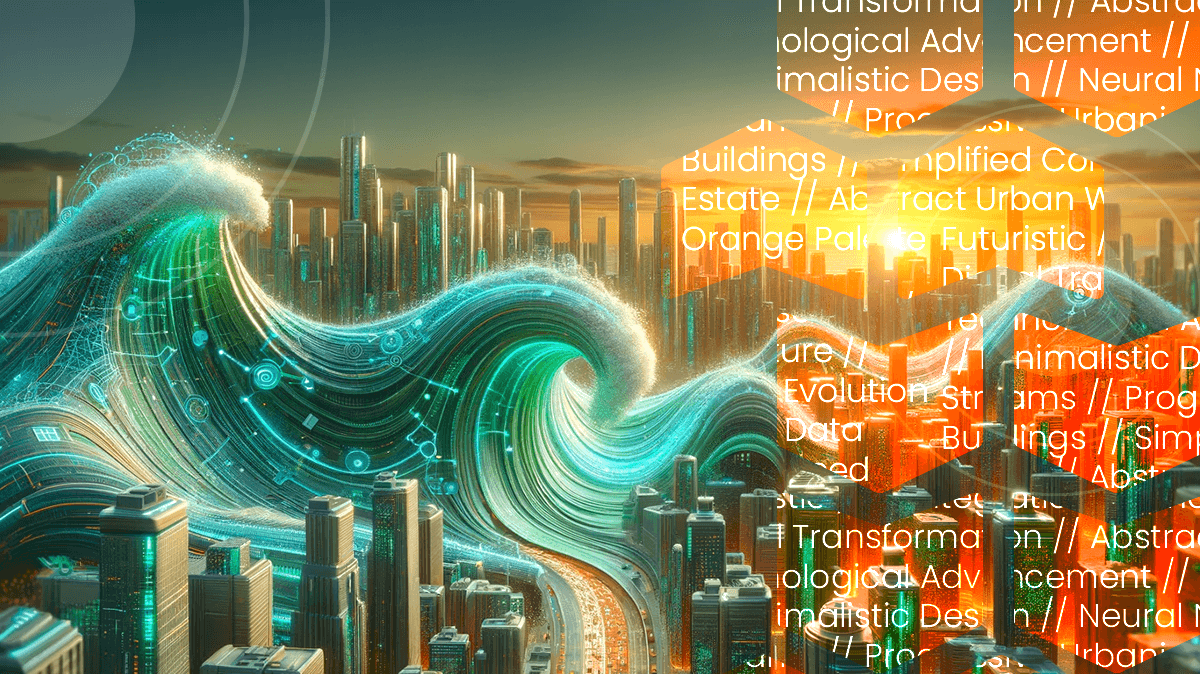
By Vin Vomero, CEO FoxyAI
In the ever-evolving landscape of enterprise technology, the emergence of Generative AI (gen AI) stands out as a beacon of transformative potential, especially in industries traditionally slower to adopt new technology like real estate.
McKinsey recently published an article that underlines the critical juncture at which the real estate sector finds itself, poised to leverage gen AI in a myriad of ways. With vast amounts of data on properties, markets, and consumer behaviors at their disposal, real estate companies are uniquely positioned to harness gen AI for tasks such as rapid identification of investment opportunities, innovative building designs, creation of marketing materials, and enhancing customer experiences. This not only promises to streamline operations but also opens up new revenue streams, painting a picture of a sector on the brink of a tech-led revolution.
However, the road to integrating gen AI in real estate is not without its challenges. Despite the excitement surrounding its capabilities, many organizations struggle with implementing and scaling gen AI solutions effectively. It’s not as simple as adopting off-the-shelf models; a deep understanding of the technology, coupled with strategic organizational changes with enterprise specific data and industry expertise is crucial.
While the entire 3,500 word article is worth the read, several key points stood out as particularly important. The article also shared some great use cases and insights into how companies have benefited from their work with AI. I will highlight some of these and add to them based on our own experiences as an industry participant.
An important theme throughout the article is that it’s “not as simple as just deploying one of the major foundational models…”. When large language models (LLMs) like ChatGPT arrived, many thought it was going to be a cure-all – one-stop shop for anything and everything AI. After playing with ChatGPT, many realized that while it worked well for broader use cases, e.g., writing a draft blog or creating general purpose generic marketing materials, it failed to produce consistent results on domain specific information and would “hallucinate” or fabricate information when it wasn’t available. Fabrication of information is clearly an unwanted and unintended output that can lead to the spread of misinformation. As discussed in an article published in Towards Data Science, “Such hallucinations happen because LLMs are trained on data that is often incomplete or contradictory. As a result, they may learn to associate certain words or phrases with certain concepts, even if those associations are not accurate or are unintentionally “overly accurate” (by this I mean they can make up things that are true but not meant to be shared). This can lead to LLMs generating text that is factually incorrect, inadvertently overly indulgent, or simply nonsensical”.
For those more familiar with AI, this wasn’t unexpected. Even before LLMs, most AI models were fine-tuned with domain or use case specific data on top of larger pre-trained models. These models came out of university from pioneers like Geoffrey Hinton and Fei Fei Lee, or were built by the likes of Google, Microsoft, Amazon, etc., just like the gen AI models that are so popular today. In the same way, LLMs must be fine-tuned on complete and accurate domain specific data in order to see the desired results. In a nutshell, ‘the garbage-in garbage-out theorem’ still applies.
Another important topic is the new role of a prompt engineer and creating a prompt library. In practice, LLMs are prompted with questions you want it to answer, or given instructions on how it should act. For example, “Use the following resident history and property data to craft an initial outreach email to a resident looking to renew their lease.”
These prompts can be quite finicky. Even changes to one word can result in different responses. The McKinsey article states: “Slight edits in syntax, detail, or framing can yield meaningfully different outputs with an impact that can only be discovered in action. There is no precedent for knowing what works until it is tried. … a rigorous process of testing and refining to ensure questions return expected answers is essential.”
Creating a library of tested prompts to be utilized by the end users or turned into API endpoints for programmatic use is vital to the project.
Lastly, McKinsey proposes enterprises take a 2×2 approach to implementing AI projects. “Identify two use cases that can launch a company into taking ownership of data, deliver measurable impact quickly, and build excitement; and identify two use cases that are more aspirational, will fundamentally change the business, and take more time to deliver. This approach encourages companies to push the technology toward its full potential.” I really like this suggestion, however, at FoxyAI we tend to limit this approach to larger enterprises with the resources to invest in several projects at once. In our experience, we have found that a 1×1 approach – one use case that will deliver immediate value and one use case that is a moonshot – is better suited for small to mid-sized companies.
In summary, the integration of generative AI into the real estate sector is not merely an advancement, but a paradigm shift, poised to redefine how the industry operates. The industry will need to quickly adapt and iterate on the transformative advances that come with the new horizons of AI. The insights from McKinsey’s article, along with practical experiences, highlight that the journey towards effective gen AI implementation is intricate and nuanced. While foundational models like ChatGPT offer a glimpse into the potential of AI, their true efficacy in domain-specific applications hinges on meticulous fine-tuning and strategic application. The evolving role of prompt engineers and the importance of a prompt library further underscore the need for a tailored approach. It is mission-critical to ensure that the larger execution risk is managed during the infancy of this exciting new era of technological breakthroughs. Whether adopting a systemic AI strategy for your organization, a 2×2 strategy for large enterprises, or a more focused 1×1 approach for smaller companies, the goal remains the same – leveraging AI to not only streamline operations but also to effectively and accurately explore transformational customer-centric innovations in real estate.
Sources:
“Generative AI can change real estate, but the industry must change to reap the benefits”, by Matt Fitzpatrick, Vaibhav Gujral, Ankit Kapoor, and Alex Wolkomir. Published McKinsey.com, November 14, 2023.
“Understanding LLM Hallucinations: How LLMs can make stuff up and what to do about it”, by Frank Neugebauer. Published in Towards Data Science, May 8, 2023.